Bioinformatics for Bioengineering
We seek to reveal the principles of life through large-scale data analysis based on machine-learning and bioinformatics approaches, and to use these rules for designing and controlling biomolecules.
Machine-learning-guided design of biomolecules: proteins, mRNAs, promoters
The development of functional proteins such as antibodies and enzymes involves trials-and-errors where a lowly-functional wild-type protein is to be improved by random mutations. To accelerate this process, we proposed a machine-learning method based on Bayesian optimization that predicts mutations for improving the protein function to the desired direction. In the collaborative work with experimental biologists, we demonstrated that our method successfully altered the green fluorescent protein (GFP) into the yellow fluorescent protein (YFP). Recently, we also develop methods to design mRNA codon sequences for improved translational efficiency, and to design promoter sequences for improved transcriptional activity.
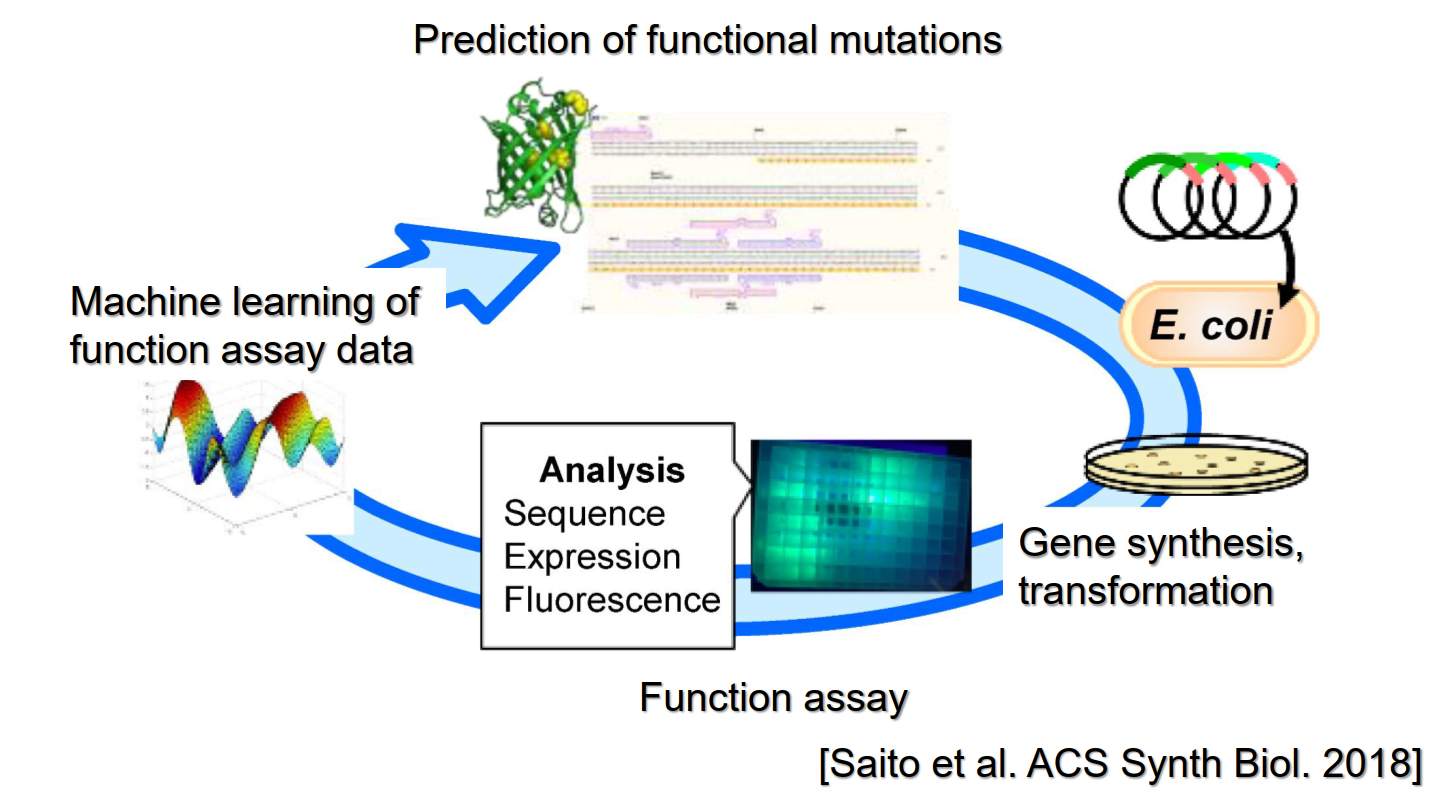
Omics data analysis: from DNA methylation to 3D genome structure
We develop bioinformatics methods for omics data analysis. Examples are: BPLA Kernel that predicts noncoding RNAs from sequence data, ComMet that detects differentially methylated regions from DNA methylation data (bisulfite-seq), and Cosearge that detects spatial co-localization of genomic elements from 3D genome structure data (HiC-seq). We also apply these methods in the collaborative work with experimental biologists: BPLA Kernel was used to find snoRNAs in the worm genome, and ComMet was used to analyze epigenomic changes in fat differentiation. Recently, we also study DNA methylation in industrially-important microorganisms.
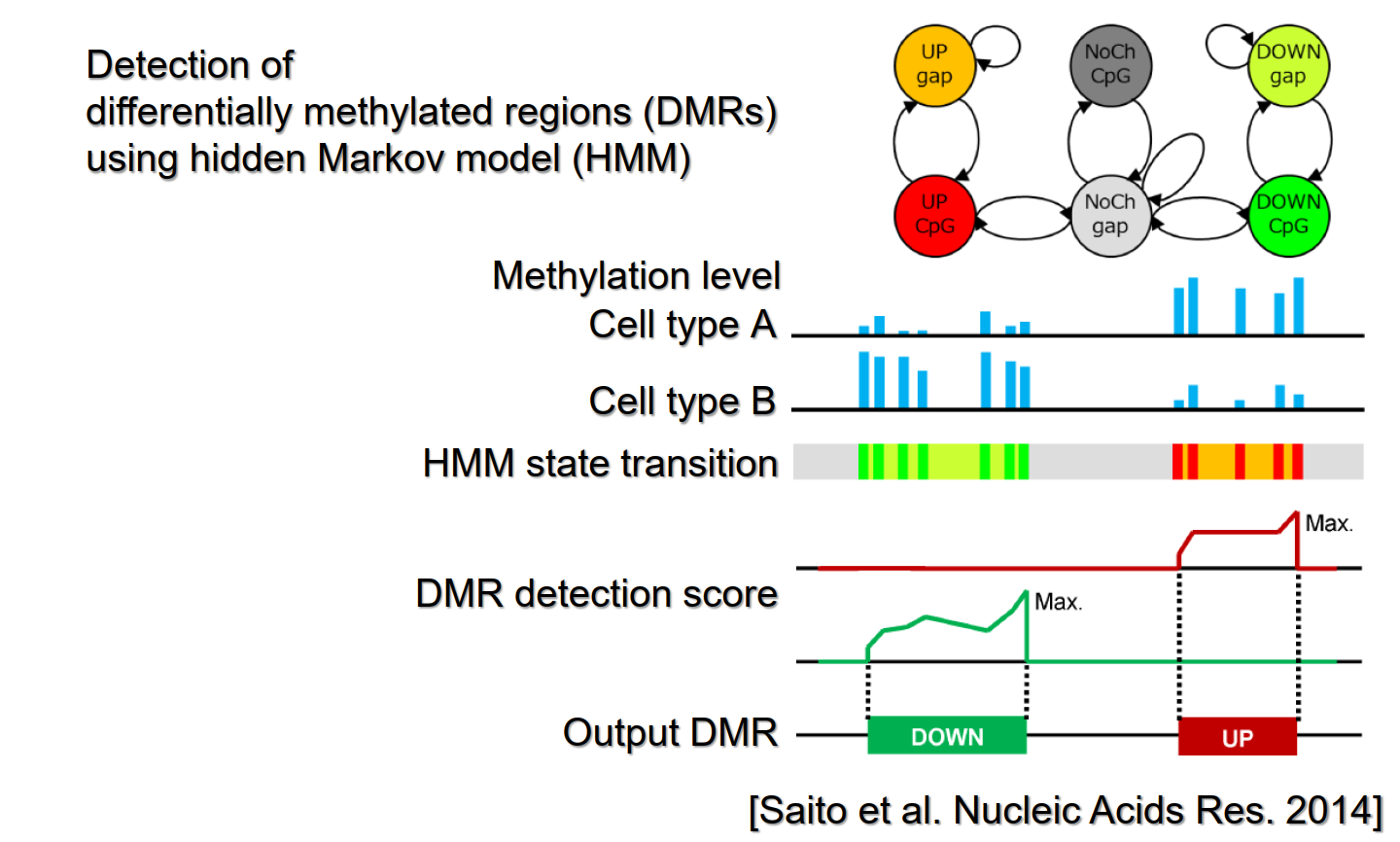